AI does not mean unbiased
While AI can be unbiased, it is generally safe to assume that it will be biased unless proven not so. There are many reasons why that is.
Published on 2023-09-13 | 3m 23s
As I was browsing through Facebook because I was looking for a certain post, I came across this post that immediately had my eyebrows up.
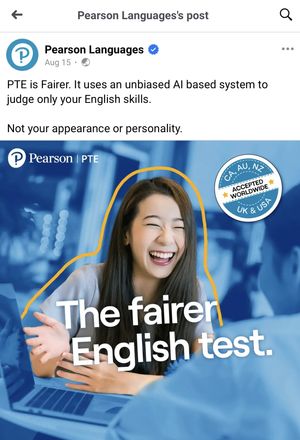
When talking about the dangers of AI, people tend to focus on hypothetical scenarios such as "They will create killer robots". While these have merits, the conversations usually ignore the risks and dangers that already happened. And this ad is one of them: Assuming the machine is unbiased. In fact, it can be much worse than humans.
Here's an example. Here's another. Here's another. And another. And more. And more. And more. You can even just scroll through this incident database (though not all of it is due to bias).
I won't say it isn't impossible to create an unbiased AI. But I will say it is a huge claim to make that requires a lot of proof. It's not something that should be thrown around lightly unless you are 100% sure it is because most of the time, it is not.
Think of the Data
To understand why this is, you must understand that AI is just a computer finding patterns from given data. And that's the danger - it's finding patterns from given data. This makes the data you use to train the AI incredibly important.
So if Pearson is going to claim that their model is unbiased, they need to show that the model used unbiased data for its training. Because if they don't, they risk perpetuating stereotypes. In fact, as it is trained on more data that is biased, it could end up performing worse than a similar person if that data is also flawed.
If the given data used to train the model is biased towards American accents, then there's a good chance the AI will have the same bias. So even if you have decent English skills (just with an Australian or Indian accent), there's still a chance that it will fail you because you are "wrong".
But even if we assume that it doesn't actually listen to intonation but rather the content itself, it is still problematic. There's a thing called dialects. So imagine if it was biased towards a certain dialect. You could fail because you used the "wrong" terms. An example is using the word "bathroom" instead of "toilet" despite the two being used interchangeably in your hometown. Or "takeout" instead of "to go". Or "pharmacy" instead of "drugstore".
There is even a problem of cultural context. For example, "nosebleed" in the Philippines doesn't usually mean an actual nosebleed. Rather, it is usually said to mean, "I have a hard time understanding."
So when Pearson says that the English test is not biased, did their data include these cultural nuances? The dialects? How about various age groups? There are words and intonations the younger generation uses that the older generations will scoff at. And there are words that mean different things to different generations as well. Did the data include these?
We're not even going to disabilities. Some people stutter - that isn't an indication that someone isn't fluent. Some people mix up certain words when speaking but are perfectly capable of understanding and speaking the language.
Language is also nuanced and evolving. A model trained on data from two years ago could fail a lot of fluent people today simply because it isn't speaking the same English from a few years ago. How is the model to account for that?
Conclusion
I'm not gonna say that Pearson's claim is false, but I do want evidence that it is true. They say their test is "fairer". Compared to what? A human proctor? If so, what is the data trained on it? How did they test it? A person can clarify what the other person meant if there was a misunderstanding. Can the AI do so?
I understand that humans are biased, and many people got frustrated at failing an English test because of things outside of their control. But I also think that claiming a machine is not biased without providing evidence is irresponsible.
P.S. For more reading, you can read this article from WeForum on how to make AI less biased.